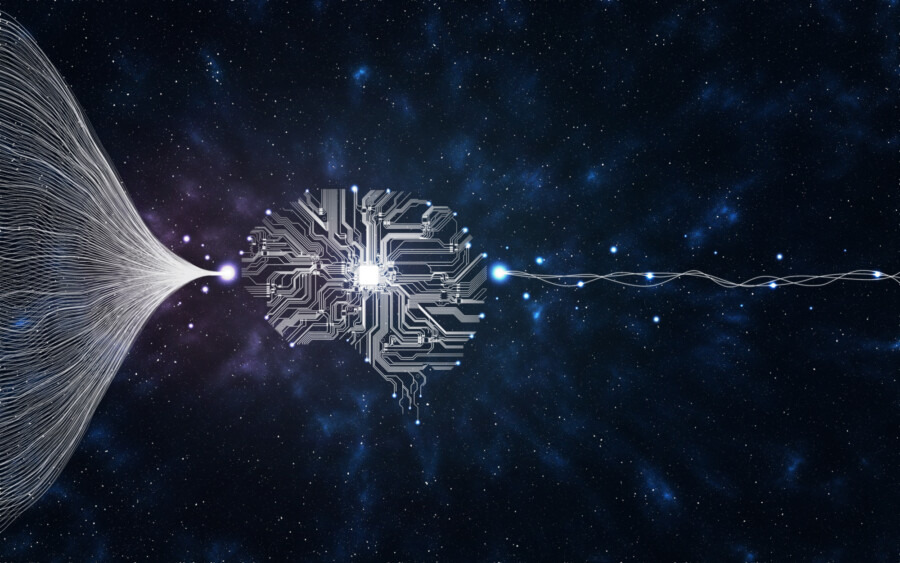
A few years ago, artificial intelligence (AI) was still ahead of us and “in the future.”
And yet, in today’s world, we see machine learning (ML) utilized everywhere. Almost all of us use this technology several times a day without even thinking about it. The range of areas where machine learning can be leveraged is nearly endless.
Speaking from my experience in the utilities industry, utilities around the world are now actively looking for opportunities to adopt AI. Other industries are following suit. While AI is not new, it’s easier to use than ever, given the prevalence of much more data combined with more affordable processing power.
What’s the difference, though, between artificial intelligence and machine learning? And what, for that matter, is deep learning?
Though clarity is key to successful innovation, these terms can be used loosely in conversations around the topic of artificial intelligence. In 2016, when Google DeepMind’s AI-empowered AlphaGo computer program took on Korean Go master Lee Sedol in a five-game match, media coverage used AI, machine learning, and deep learning interchangeably—as if they all referred to the same type of intelligence.
Let us discuss these terms separately, so that we can speak clearly and accurately on this subject of emerging importance.
What Are Artificial Intelligence, Machine Learning, and Deep Learning?
Artificial Intelligence is the broadest and most all-encompassing of these terms, having been coined as early as 1956, when the field of AI research was founded at a summer workshop at Dartmouth College. It refers, generally, to the ability of machines to exhibit human-like intelligence.
In studying AI, researchers often distinguish between narrow AI, or the ability of technology to simulate human intelligence in focus on one narrow task, such as playing chess, and artificial general intelligence (AGI), a still-hypothetical type of intelligent agent that is level with (or superior to) human intelligence in the majority of economically valuable tasks.
AI encompasses several different technologies and systems. Machine learning is one of these branches of AI; others include natural language processing, computer vision, and speech recognition.
Machine learning refers to the use of algorithms to parse large volumes of data, learn from the process of doing so, detect patterns, and then make decisions or predictions based on these patterns. It’s so named because it imitates the way that humans learn, gradually improving its accuracy through accumulated experience.
Deep learning is a subset of machine learning. It is inspired by neural networks in the human brain and is generally understood as a method for implementing machine learning, equipping artificial neural networks with a set of techniques known as representation learning, which automatically discover meaningful patterns from raw data. Recently, deep learning has proven highly successful. Again, it is dependent on massive datasets to “train” itself.
Why AI, and Why Now?
Since it was first conceptualized, artificial intelligence has seen its fair share of ups and downs, of ebbs and flows in public interest. But today, we’re finally seeing real progress, and AI is set to transform our world. The main reasons for this are:
- Massive computational power is now available at a low cost and can be provisioned quickly in the cloud. Improvements in graphics-processing-unit (GPU) computing have increased the training speed of deep-learning algorithms, given that graphics cards now feature thousands of cores and are therefore ideally suited to parallel workloads.
- Big data has exploded in recent years, with a massive increase in the amount of data we all create coupled with near-limitless storage capacity. Large and diverse data sets provide better training material for algorithms.
- Algorithms themselves are now better at finding patterns in the mountains of data. AI and machine learning platforms from players such as Google, IBM and Microsoft are making it much easier to develop powerful applications that support and enable these algorithms.
The technology world’s investment in AI, particularly in the areas of machine learning and deep learning, is growing at a rapid pace. Machines are already as competent as humans, if not more so, at specific tasks: playing chess, transcribing audio, analyzing images, and diagnosing diseases.
However, adoption of AI across business processes at the modern enterprise remains low. Business leaders are uncertain about what capabilities AI can truly unlock for them, where to obtain AI-powered applications and how to integrate them into their companies.
To that end, when should business leaders consider machine learning?
Machine learning is suited to business problems where:
- The decision or prediction to be made is complex in nature (for example: face detection, speech recognition, spam filters),
- The organization has access to high-quality, clean, and recent or real-time data, ideally labelled to allow the algorithm to make sense of it.
- Some margin of error is acceptable.
AI opens a wide range of exciting possibilities for all industries, but the changes will not be simple.
Early evidence suggests AI can deliver real value to serious adopters, but current uptake outside of the tech sector remains low. Few companies have deployed it at scale. As with all such innovations, opportunities and rewards still await those willing to take the plunge.
James McClelland is responsible for global marketing for the utilities industry at SAP. Learn more about this topic at SAP for Utilities, Presented by ASUG (October 9-11, 2023) in Chicago, IL.